Patent Mining: Unlocking the Latent Value of your Patent Portfolio
Despite the disruption caused by the COVID-19 pandemic, the number of patent filings has been on an upswing. Almost 3.3 million patent applications were filed in 2020, recording an increase of 1.6% as compared to 2019. This extensive patent data presents an unprecedented opportunity to derive actionable business insights out of it. Similar to the concept of data mining, we also have patent mining to make sense of patent data. Simply put, patent mining is the act of mining specific patents from an extensive database. It entails identifying IP assets to license, sell, abandon, or acquire to enhance the portfolio’s efficiency. Patent mining is done with business goals in mind and could be performed manually, or automatically with state-of-the-art tools.
This article will discuss the concept of patent mining, its need, several tasks involved, and more.
Table of Contents
What is the Need for Patent Mining
The five basic needs for patent mining are shown in Figure 1.
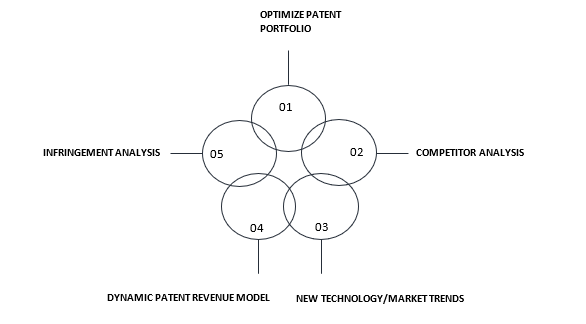
1. Optimize Patent Portfolio: Optimizing a patent portfolio is a significant problem for organizations investing in a continuous stream of new patents. Identifying the patents of interest in a large patent pool requires specialized skills and experience. Patent mining has emerged as a tested tool to address this challenge. There could be several benefits of patent portfolio optimization, including pruning for high-value patents, finding technology-relevant patents, or discarding patents that seem to exhaust an organization’s resources.
2. Competitor Analysis: Although there are new and old techniques for conducting competitor analysis, patent mining is more ingenious and reliable. Digging into a competitor’s patents can reveal exciting insights into your adversaries’ direction, technology, and consumer-friendly features. Besides, by analyzing the competitor’s patents, a company can develop a strategy that may help it stay ahead of the curve in the future.
3. Understand New Technology Trends: The technology landscape has become steep in the last decade or so. Understanding the existing and emerging technologies is imperative to make a mark in a new market. The system of patent mining facilitates technology road mapping. The technique helps to compare the stage of technological maturity accomplished by the organization vis-à-vis its competitors dominating the technology. Moreover, an organization can leverage the information to explore new technical partnerships, technology transfers, or even the acquisition of a start-up.
4. Create Dynamic Patent Revenue Model: Patent mining enables a business to identify patents strategically essential for other players in the market. Thus, the exercise allows the monetization of old patents by selling or licensing them to create a dynamic patent revenue model. Since technology penetration is not always swift, patents related to relatively obsolete technology can also be valuable for some organizations in an industry.
5. Patent Infringement Analysis: Conducting infringement analysis on an exhaustive set of patents is both a time-consuming task and an out-of-date approach. Patent mining helps to narrow down the datasets based on their relevancy. Once the dataset is identified, patents are ranked and classified into different batches. The size of a single batch could range from one hundred to five hundred patent families. Patents with broad claim scope, low or negligible detectability issues, and a higher likelihood of infringement are marked as ‘T1’. This step ensures that most of the effort is invested in patents with a higher possibility of infringement.
After discussing the basic needs for patent mining, it is crucial to understand how it is used for infringement analysis.
How Sagacious IP Uses Patent Mining for Infringement Analysis
There are five significant steps for a compelling infringement analysis in Patent Mining. These are shown in Figure 2.
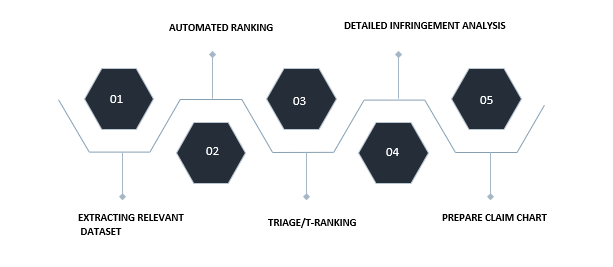
1. Extracting Relevant Dataset
The first step in patent mining is to extract the relevant patent dataset as per the client’s requirement. For instance, the need could be to identify patents relating to a specific market segment, technology segment, or a company. In certain cases, it could just be an exemplary market product.
An exhaustive list of relevant classes and keywords is identified, which is used to extract a broad set of data. Assignee and inventor-based search is also performed to extract the dataset.
Ensuring the usage of the correct database to extract data for jurisdiction-wise exhaustive coverage is also an essential factor.
Basis the size of the extracted dataset (and the client’s budget), quick manual filtering is subsequently performed to remove the irrelevant data or the false positives. The step provides a much more relevant dataset to work with before moving to the next step, i.e., automated ranking.
2. Automated Ranking
After identifying the patent dataset, the next step is to perform an automated ranking of the overall dataset. Here, patents within the dataset are relatively ranked based on objective parameters. These parameters include the number of forward citations, number of backward citations, claim count, pending life, big assignee count, number of family members, independent claim length, prosecution time, etc. In addition, in case where the objective is to identify relevant patents against a particular target company (say, X), the factor “forward cited by X” is also considered for ranking the patents.
Though citation analysis helps identify high-impact patents, as raw citation counts tend to favor older patents, it is important to compare patents against all U.S. patents from the same patent classification and issue year, in terms of the number of citations received.
The automated ranking aims to segment the comprehensive list of patents into smaller batches and prioritize a set of patents that has a higher likelihood of infringement for analysis. The action helps deliver a better outcome in less time.
3. Triage/T-ranking
After the automated ranking is complete, patents ranked higher in the list are prioritized for analysis.
For each batch of patents (batch size could be 100-500 patent families), manual analysis is performed where we invest some time in quickly reviewing the title, abstract, and claims. Then, basis the claim scope, likelihood of infringement (for example, in general, or against a particular product category), and infringement detectability, patents are ranked as T1, T2, and T3. While performing such analysis on a patent family, claims of parent patent as well as continuation, and divisional applications are also considered to identify the broadest set of claims in the family. Patents with broad claim scope, low or negligible detectability issues and higher likelihood infringement are marked as T1.
4. Detailed Infringement Analysis
At this stage, a more deep-dive review of the patent specification and file wrapper is performed to understand the patent’s novelty. The patent specification also provides cues on each patent’s possible areas of application.
We select the broadest independent claim for each patent for performing the analysis.
Once the market areas or applicable product categories are identified for a patent, the top players by revenue/popularity are identified and analyzed for the concerned market segments/product categories. The intent is to identify companies with high revenues as the infringing targets.
For a given target or company, we try to analyze documentation (product manuals, datasheets, source code, research papers), demo videos, etc., as published by the company itself (as it is more credible) to assess the infringement potential. If required, we also rely on credible third-party sources to understand the product functionality/features, etc., to ascertain infringement.
In scenarios where reverse engineering is required to showcase mapping for one or more claim elements, we try to identify as much evidence from public documentation as possible, and advise clients on reverse engineering accordingly.
5. Claim charting
Once we have identified infringing targets, we prepare a claim chart for the specified targets.
Depending on the client’s budget and on the end objective, we prepare charts with different levels of resolution. Suppose the aim is to merely conduct soft/high-level negotiations with a particular defendant for an out-of-court settlement, then we prepare one or two slider charts where concise/high-level mapping is presented to showcase infringement. However, for cases where litigation is to be initiated, we prepare in-depth or high-resolution charts where the features of the claim are broken to the nth level, and evidence for every claim clause is presented in a detailed manner.
Besides, corresponding excerpts from the specification are also presented to showcase how the patent specification supports the alleged infringement.
The analysis comments are also provided for each claim element to narrate how they correlate to the corresponding product functionality.
Color coding of claim elements is a preferred practice where each claimed feature is provided a unique color. The corresponding evidence is also highlighted with the same color for ease of product to claim correlation.
Now that we have discussed the need for patent mining and how Sagacious IP uses patent mining for infringement analysis, we will turn our focus towards patent mining tasks and techniques that produce the best results.
What are Patent Mining Tasks and Techniques
A patent document contains both structured and unstructured data. Structured data are consistent semantics and formats (patent number, filing date, inventor, issued date, and assignees). Unstructured items, however, contain text content that is different in length (such as claims, abstracts, and descriptions of the inventions). While this well-defined structure of patent documents aids in analysis, patent mining remains a challenge. This is where the patent mining tasks and techniques come in. While tasks are specific pieces of work that need to be carried out to complete the process of patent mining, techniques are ways in which a task can be completed. You can check out various patent mining tasks and techniques in Table 1 below.
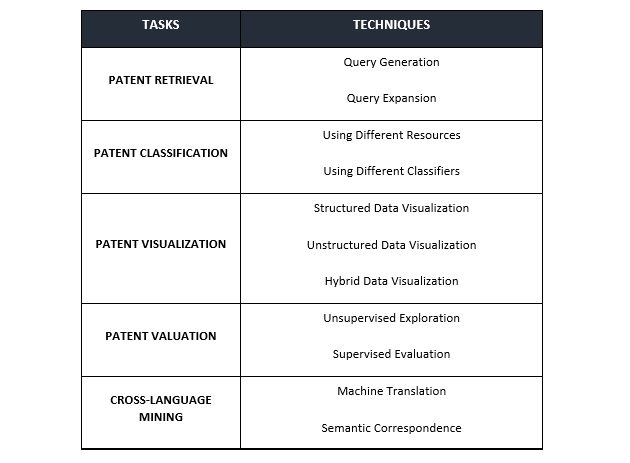
1. Patent Retrieval: The two most critical techniques for patent retrieval are query generation and query expansion. A typical procedure for patent search and retrieval can be divided into four steps as shown in Figure 3.
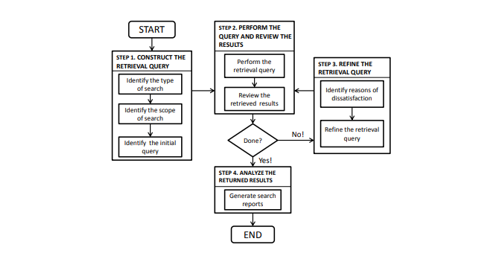
Step 1
Construct the Retrieval Query: The initial task is to determine the kind of patent search depending upon the objective of patent retrieval. Afterward, the search scope is identified, and a search query is constructed.
Step 2
Execute the Query and Review the Results: In this stage,the query constructed in Step 1 is performed. First, the users receive the results with relevant documents. Then the user verifies the returned results to decide whether the documents are as desired or not. If the results are favorable, the user jumps to step 4. Otherwise, they move to step 3.
Step 3
Refine the Retrieval Query: If the user is not satisfied with the results of executed queries (i.e., there are very few results, too many documents, or unrelated documents), they must refine the search query to obtain better results. The user can put extra constraints in the query to trim unnecessary documents, remove additional restrictions to get more results, or replace the keywords for relevant results.
Step 4
Assess the Returned Results: Once the user has carefully reviewed the returned documents, they draft a search report depending on the task in compliance with the patent law and regulation. The search report usually contains – a synopsis of the invention, classification codes, retrieval tools and databases for search, pertinent documents, query logs, and retrieval conclusions.
Despite the progress in patent retrieval tools and techniques, the task is still challenging from many perspectives. Therefore, it is crucial to understand some common obstacles before proceeding with patent retrieval. These are mentioned in Table 2 below:
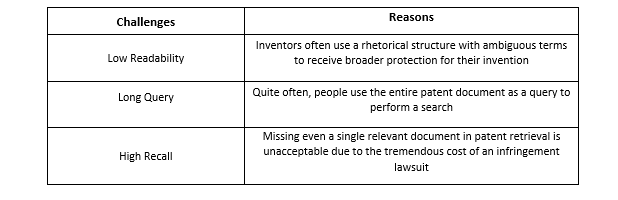
2. Patent Classification: The second task involved in the patent mining process is patent classification. There are numerous challenges to it. Three important ones have been listed below.
- Patent documents contain complicated structures, loquacious pages, and rhetorical descriptions, adding to the difficulty in extracting valuable features. This makes automatic patent classification a challenging task.
- The hierarchical structure of the patent classification outline is complicated, with an estimated 72,000 subgroups in the bottom level of IPC taxonomy.
- The large volume of patent topics and their increasing variety aggravate the hardship of automatic patent classification.
Two effective techniques have been developed for better patent classification to overcome these challenges:
- Using Different Resources: The bag-of-words (BOW) model is frequently used to represent unstructured text documents. This model selects terms and phrases based on structural information and frequency to represent patent documents. Afterward, the Vector Space Model, Naïve Bayes, and KNN (K-Nearest Neighbor) classification models are used to categorize patent documents. The performance of the KNN-based classifier is usually better than the Naïve Bayes in patent classification. The soaring popularity of the BOW-based representation stems from its simplicity. However, it is challenging to demonstrate the relationship among terms using the BOW-based model. But the issue can be addressed using sematic structural information that facilitates patent classification. The sematic structural information consists of six tags: technology field, claim, purpose, method, explanation, and example. First, a new representation is generated for a given patent document based on the semantic tags. Then the similarity is ascertained based on semantic tag and term frequency. Eventually, the KNN-based model is employed to classify patents automatically. Not surprisingly, the approach has achieved an improvement rate of 74% in Japanese Patent Classification.
- Using Different Classifiers: The classification accuracy varies depending on the algorithm used and parts of a patent document (title, claim, and first three-hundred words of the description) utilized. For example, Support Vector Machine (SVM) produces the best result for class-level patent document categorization. It utilizes the first three-hundred words of the description for demonstrating patent documents.
3. Patent Visualization: It is the third task in the patent mining process. The complex structure of patent documents limits the capability of an analyst to comprehend the core idea of the patent. The solution to the problem is patent visualization, which helps quickly identify the correlation between different patent documents. The three ways of patent visualization are listed below:
- Structured Data Visualization: In this way of patent visualization, structured data, including patent number, issued date, filing date, and assignees, is utilized to produce a patent graph. The primary resource used for graph generation is the citation information in different patents. The citation graph makes it easy to identify interesting patterns concerning specific patent documents. An example of structured data visualization is shown in Figure 4.
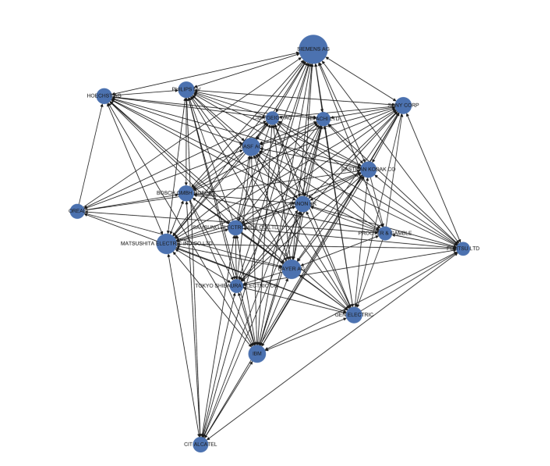
Here, the 20 nodes represent 20 prolific companies in terms of patents while each edge represents a citation between them. Each node’s size is proportional to the number of citations received by it.
- Unstructured Data Visualization: This helps in reducing domain knowledge dependence. Unlike citation analysis, the content-based patent map has significant benefits in extracting latent information and global technology visualization. We can generate several kinds of maps from the unstructured text in patent documents, including technology vacuum map, technology portfolio map, and claim point map. An example of unstructured patent data visualization is shown in Figure 5.
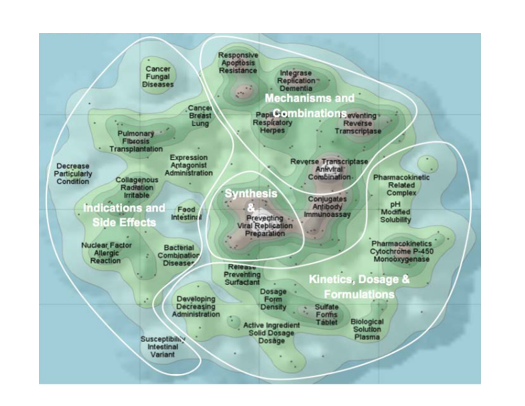
- Hybrid Data Visualization: Structured and unstructured data are often combined for better visualization. Unlike the conventional technology vacuum map built entirely on patent content, the hybrid approach combines bibliographical information, such as file date and assignee information, to generate the patent maps. As a result, it facilitates better comprehension of technology trends in shaping R&D strategies.
4. Patent Valuation: This is the fourth task in the patent mining process. The practice and procedure of evaluating the relevance/quality of patent documents is defined as patent valuation. The aim is to aid internal decision-making pertaining to patent protection strategies. One example is the collection of related patents called patent portfolios to form a super patent pool. However, the pressing question is how to calculate the potential benefits of patents to select the most crucial ones. The solution to this issue is utilizing two patent evaluation techniques – Unsupervised Exploration and Supervised Evaluation.
5. Cross-Language Mining: The primary aim in cross-language patent mining is cross-language information retrieval with the query written in a familiar language. In general practice, a cross-language patent retrieval system is constructed using two techniques – semantic correspondence and machine learning.
Conclusion
We can conclude from the above-mentioned details that patent mining can unlock the tremendous potential of a large pool of patents. However, a desirable result is subject to the condition that the above-discussed tasks and techniques are well executed. Apart from infringement analysis, patent mining is also helpful for other types of search and analysis – prior art search & analysis, patentability search, invalidity search, legal status search, etc. All search and analysis results provide a competitive edge to a business. Although patent mining tasks and their techniques can be performed independently by an organization, it is advisable to avail the services of a reliable law firm with expertise in the field.
Sagacious IP has carved a name for itself through twelve years of distinguished service. It has delivered more than 25,000 projects globally with four delivery centers and offices in six countries. In addition, the company has acquired an enviable clientele by serving more than 5000 clients with next-generation IP solutions. Moreover, through our Patent Mining services, we help clients identify technology to license or sell marginal assets to generate revenue, donate or even abandon assets to reduce costs, and identify acquisitions to strengthen or expand an existing portfolio. Click here to know more about this service.
-Abhinav Mahajan (ICT Licensing) and the Editorial Team